After Many Years Of Talk, Artificial Intelligence Is Coming Into Its Own As A Powerful Solution To Cement’s Many Challenges.
By Jonathan Rowland
Artificial Intelligence (AI) has received a lot of attention of late in a broad range of industries, including cement. So much so, that it’s easy to think of it as something new, innovative – 21st century. But as a concept, it’s been around since the mid-1950s. It was, however, an idea ahead of its time: the technology simply didn’t exist to make it a reality.
Almost seven decades later, much has changed. AI is becoming an almost ubiquitous part of modern life: from satnavs to Alexa and Siri, Amazon and Netflix to high-speed stock trading and weather forecasting, the uses of AI are all around us.
“AI has become more than just a ‘thinking machine’ buzzword from computer science,” Sanjit Shewale, head of digital for process industries at ABB, told Cement Americas. “We see its use accelerating and expanding across process industries.”
According to Shewale, the growth in AI is driven by three main developments:
1. Expanding computational power and increased training speed of deep neural networks.
2. The availability of connected devices and accessibility of endless data storage via the Cloud.
3. With access to more data, algorithms are getting better at finding patterns and new optimization opportunities.
In the cement industry, AI offers the chance to come at age-old challenges with a fresh perspective: reducing the cost of operations, while maximizing yield, improving quality and reducing emissions at the same time. It’s a balancing act to which AI is ideally suited, as Liran Akavia, COO and co-founder of Seebo, a company that specializes in process-based AI, explained.
“AI offers the ability to achieve global, continuous optimization across multiple KPIs – many of which appear to conflict with one another – rather than just local optimization,” said Akavia. “Cement manufacturing processes are very dynamic, even temperamental; AI can look comprehensively at the entire process, and recommend the optimal process settings and set point to take the entire production line – across all its complexities, interrelated tags and conflicting KPIs – to the next level of efficiency and profitability.”
AI is a “gamechanger,” agreed Shewale. “The ability to perform advanced data analytics and smart optimization, powered by AI, allows cement producers to hit KPIs around sustainability, process and asset performance, connected workers and operational excellence.”
Herding Cats?
It is this ability to understand the dynamic conditions inside a cement plant that gives AI its value. As Seebo’s Akavia noted, optimizing cement production – with its multiple KPIs, many dynamic inefficiencies and countless external influences on the production process – can “often feel like herding cats.”
“By contrast, machine learning (ML) algorithms are capable of conducting continuous, multivariate analysis of exactly this kind of process, which is why ML is the only path to truly mastering the cement production process,” continued the Seebo co-founder. “One major caveat is that the algorithm must have a deep understanding of the context behind the data; namely, the highly complex and dynamic nature of the production process.”
It’s a theme that ABB’s Shewale also emphasized: “successful ML solution implementation requires standardized data, with a data management approach closely aligned to the business strategy. The best classification model requires many iterations and a rare combination of data science, very specific cement industry expertise and ingenuity. To optimally operate assets and dynamic processes, it is important not to treat them separately.”
“Of course, it is impossible to achieve 100% efficiency, no matter what methods and tools you use,” concluded Akavia. “But this path can bring cement manufacturers as close as possible to total efficiency.”
The Benefits of AI
So far, generally speaking, what then are some of the tangible advantages AI can deliver by herding those proverbial cats? For Seebo’s Akavia, the major advantage of AI is the ability to “reveal the hidden causes of production losses throughout the entire process – from the raw materials to 28-day strength – by providing end-to-end insights and recommendations on how to prevent those losses, in areas such as clinker quality, kiln throughput, energy efficiency and emissions.”
Improving energy management. ABB’s AI-based anomaly detection app learns the “normal” operating state of plant and equipment, and then uses adaptive setpoints to detect unusual patterns and anomalous behaviors, triggering alerts when such conditions are detected. This “reduces the effort to identify and rectify energy consumption deviations,” explained Shewale. “There’s no more hassle of setting manual setpoints or alarms, no more notification overload.”
In the same way, an AI app can learn from energy usage, production schedules and other factors to deliver accurate forecasts, allowing a cement plant to reduce peak demand charges on electricity bills.
Optimizing process performance. Due to the variability in feed and fuel, coupled with the complex process dynamics, manual operators will tend to remain safely within process constraints in order to ensure against deviation from setpoints. But this comes at a cost to plant profitability. With AI-powered advanced process control (APC), however, cement plants can maximize performance, without overstepping limits, e.g., on allowable emissions levels.
“ABB’s APC solution is being successfully used to address a range of process performance issues,” said Shewale. “For example, increasing feed by over 3 tph, while reducing specific energy by 20 kcal/kh; achieving zero SO2-emissions violations, while reducing hydrate consumption; and simultaneously delivering overall productivity increases and more consistent cement quality.”
Using ML with data-driven soft sensors, cement plants are also now able to predict 28-day strength on the day of sampling, allowing for timely and precise process corrections e.g., setting new daily CaCO3/Blaine targets. As a result, more cement will be sold at correct specification, reducing the need for additives.
AI for asset management. Predicting how cement plant assets will react to triggers such as age or operating conditions is a challenge, because complex systems interact in unexpected ways and are always evolving. It’s made harder by the fact that the degree of complexity may be “beyond the human eye,” continued Shewale. “Leveraging AI for asset performance management (APM) is a step change in the way maintenance and reliability professionals can collaborate with other functions, ensuring assets are available at the time and at the performance level required by the operations, while taking into account changing production goals.”
“To provide accurate target parameter predictions in near real-time and prevent failures, AI/ML models need to be continuously trained with relevant datasets, requiring deep understanding of both cement processes and asset behavior. Think of AI-enabled APM as the most cost-effective way to extend the life of the aging (and newer) assets, to decide on the optimal timing for scheduled maintenance turnarounds (one of the biggest costs in a plant) and make maintenance planning better.”
An Evolving Technology: Empowering Autonomy
With the increase in instrumentation, data historians and other databases, cement plants are awash with data as never before. This provides the means for AI advisory systems to further evolve and improve. At the same time, the effort involved in AI implementation is decreasing. And then there are tightening sustainability targets, which will be impossible to reach by manual setpoint adjustment. As a result, ABB’s Shewale expects to see “more and more people get involved in AI.”
In terms of the technology, “we are going to see a lot more of unit area models: plant models able to continuously retrain themselves based on outside disturbances. This will give confidence to cement companies, making them more comfortable with the idea of autonomous operations. I do still think that core solution areas, such as operational excellence, process performance, asset performance, and others are going to be needed. These are core fundamental areas that will continue to provide value, but these types of solutions can become autonomous themselves. To me, that’s what AI is going to bring.”
AI-powered advanced process-control systems, such as ABB Ability Expert Optimizer, can assist cement operations achieve their optimum state. PHOTO: ABB.
Busting the Jargon
The world of AI is adrift with jargon that can easily take out the unsuspecting traveler. ABB’s Sanjit Shewale provides a guide to some of the more common terms.
Artificial intelligence (AI) is like a formula that has the ability to achieve set goals in new situations: i.e., the formula can adapt to change rather than remaining a static algorithm.
Machine learning (ML) is a subset of AI. It is the principle that a machine can learn without human intervention, developing its own algorithm to improve the performance of a specific task.
A neural network is a set of algorithms loosely modeled on the way the human brain processes information.
Deep learning is a more sophisticated version of ML that is used to perform more complex tasks or to produce data needed for decision-making. It uses multi-layer neural networks for a more powerful way to filter and process information.
Finally, it’s important to keep a couple of caveats in mind: first, ML is only able to solve the problems formulated for it; and second, not every optimization method it learns from the data would make sense in real-world conditions or deliver tangible benefits.
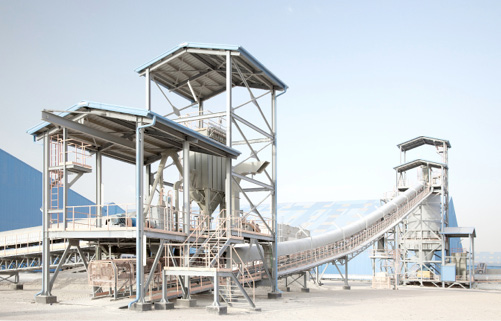
An AI Methodology
Seebo’s Process-Based Artificial Intelligence is the foundation of a tried-and-tested formula that the company uses to navigate the highly-complex environment found in the cement plant, as company co-founder and COO, Liran Akavia, explained.
“Our methodology starts with working with the plant team to set their key objectives and constraints. Inevitably, there are a lot of conflicts. Our Process-Based AI then learns the production process, analyses the data, and creates a single measure of efficiency that takes all the different objectives, KPIs and constraints into account. Once that is achieved, the algorithms deliver recommendations as to the precise combination of set points and ranges which, when reached together, will optimize process efficiency across all KPIs.”
Making this possible is an “AI that is capable of handling and optimizing multiple KPIs, rather than an AI tool that focuses on a specific KPI or an isolated aspect of your production process.”
Thinking Beyond the Cement Plant: Enterprise-Level AI
Using deep domain expertise, ABB Ability Genix Industrial Analytics and AI Suite integrates operational, engineering and business data with analytics and AI, giving the ability to make better, smarter business decisions. According to ABB’s Sanjit Shewale, such enterprise grade AI-based solutions have enormous potential for achieving operational excellence, helping companies to understand the reasons behind the differences in cement plant performance levels.
“Transferring knowledge or process methodology from the higher-performing to the lower-performing plants will optimize production and uncover best operating points to meet and even exceed KPIs,” Shewale said. “With less cement required in the future for modular prefabricated buildings, AI will also play an important role in restructuring operations to retain profitability at reduced cement demand. By analyzing how procurement was done in the past, it can also assist with better planning for supply chain management.”